Artificial intelligence (AI) and machine learning (ML) are revolutionizing cloud data management, enabling organizations to optimize processes, enhance security, and derive actionable insights. This blog delves into how AI and ML are transforming cloud data management and why these technologies are indispensable for modern businesses.
Understanding AI and ML in Cloud Data Management
AI refers to the simulation of human intelligence in machines programmed to perform tasks such as decision-making and pattern recognition. ML, a subset of AI, involves training algorithms to learn from data and make predictions or decisions without being explicitly programmed.
In cloud data management, AI and ML automate and enhance processes such as data classification, anomaly detection, and predictive analytics. These technologies help businesses manage data more efficiently while uncovering valuable insights hidden in large datasets.
Key Benefits of AI and ML in Cloud Data Management
1. Enhanced Data Organization
AI and ML can analyze large datasets to categorize and organize information automatically. This capability helps businesses:
Identify and tag sensitive data for compliance purposes.
Classify data based on relevance, usage, or importance.
Streamline data retrieval and improve accessibility.
2. Improved Security
Cloud environments face constant threats from cyberattacks. AI and ML enhance security by:
Detecting anomalies and unusual patterns in real time.
Identifying potential threats before they become breaches.
Automating responses to common security incidents.
3. Predictive Analytics
Predictive analytics powered by ML enables businesses to anticipate future trends and make informed decisions. Examples include:
Forecasting storage requirements based on historical data.
Identifying bottlenecks in workflows to optimize operations.
Predicting customer behavior to enhance service delivery.
4. Cost Optimization
AI and ML help optimize cloud expenses by:
Monitoring resource usage and identifying underutilized assets.
Suggesting cost-efficient storage solutions.
Automating workload distribution to reduce computational costs.
5. Scalability and Efficiency
As data grows, manual management becomes unsustainable. AI and ML ensure scalability by automating repetitive tasks, such as:
Data migration across cloud environments.
Managing backups and recovery processes.
Scaling resources dynamically based on real-time demands.
AI and ML Applications in Cloud Data Management
1. Intelligent Data Classification
Manually classifying data is time-consuming and prone to errors. AI-driven tools can:
Identify and label data based on its content and context.
Differentiate between structured and unstructured data.
Ensure compliance by tagging sensitive or regulated information.
2. Automated Backup and Recovery
AI and ML streamline data protection by:
Automating regular backups to avoid data loss.
Predicting potential failure points in storage systems.
Ensuring faster recovery times by prioritizing critical data.
3. Anomaly Detection
ML algorithms excel at recognizing deviations from normal patterns. In cloud data management, this translates to:
Identifying unauthorized access attempts.
Detecting unusual spikes in data usage.
Preventing potential breaches before they occur.
4. Smart Resource Allocation
AI-driven systems optimize cloud resources by:
Allocating storage and compute power based on workload needs.
Reducing costs by scaling resources up or down automatically.
Monitoring performance to avoid resource bottlenecks.
5. Advanced Analytics and Reporting
AI and ML provide actionable insights by:
Analyzing data trends and patterns.
Generating comprehensive reports for decision-making.
Visualizing data for easier interpretation by non-technical users.
Challenges of Implementing AI and ML in Cloud Data Management
While AI and ML offer numerous advantages, businesses may face challenges when integrating these technologies into their cloud data management strategies, let’s take a look at some of the challenges they may face and some possible solutions:
1. Data Quality and Availability
AI and ML algorithms require high-quality, well-organized data for effective training and operation. Poor data quality or insufficient data can hinder performance.
Solution:
Invest in data cleansing and preprocessing tools.
Ensure consistent data governance policies.
2. Skills Gap
Implementing AI and ML requires expertise in data science, machine learning, and cloud technologies. A lack of skilled professionals can slow adoption.
Solution:
Provide training programs for existing staff.
Partner with AI and ML service providers.
3. Cost Considerations
Developing and deploying AI and ML systems can be resource-intensive, especially for smaller organizations.
Solution:
Start with scalable, cloud-based AI solutions.
Focus on use cases with the highest ROI.
4. Ethical and Compliance Concerns
AI and ML must adhere to privacy regulations and ethical guidelines to avoid misuse or bias in decision-making.
Solution:
Regularly audit algorithms for compliance and fairness.
Implement transparent policies for AI use.
If businesses can adopt this listed solutions, they can greatly improve their cloud data management strategy. Next up, we look into some of the best practices in leveraging AI and ML in cloud data management.
Best Practices for Leveraging AI and ML in Cloud Data Management
1. Define Clear Objectives
Identify the specific problems you want AI and ML to solve, such as improving security, optimizing costs, or enhancing analytics capabilities.
2. Start Small
Begin with a pilot project to test AI and ML applications in a controlled environment. Use the insights gained to scale implementation gradually.
3. Choose the Right Tools and Platforms
Select cloud providers and tools that offer robust AI and ML capabilities, such as:
AWS (Amazon SageMaker, AI-driven analytics tools)
Google Cloud (BigQuery ML, AutoML)
Microsoft Azure (Azure AI, Cognitive Services)
4. Focus on Data Governance
Establish policies for data access, storage, and processing to ensure compliance and consistency.
5. Continuously Monitor and Improve
Regularly evaluate the performance of AI and ML systems to identify areas for improvement. Update models as new data becomes available.
To conclude this section, embracing these best practices allows businesses to extract maximum value from their AI and ML initiatives. A strategic approach ensures that the integration of these technologies enhances operations and drives meaningful outcomes.
Future Trends in AI and ML for Cloud Data Management
As we look ahead, several emerging trends are poised to redefine the role of AI and ML in cloud data management. These advancements promise to address current challenges while opening up new possibilities for innovation and growth. Let’s explore the trends shaping the future.
1. Explainable AI (XAI)
As AI systems become more complex, understanding their decision-making processes is crucial. Explainable AI will ensure transparency, helping businesses trust and verify AI-driven decisions while maintaining compliance with regulations.
2. Federated Learning
Federated learning enables ML models to train on decentralized data without compromising privacy. This approach ensures sensitive data stays secure while allowing organizations to leverage insights from distributed datasets.
3. Integration of Edge AI
With the proliferation of IoT devices, edge AI will play a pivotal role in processing data closer to its source. This reduces latency, improves real-time analytics, and minimizes the bandwidth required for transferring data to the cloud.
4. AI-Driven Data Lakes
Data lakes powered by AI will allow businesses to store, process, and analyze vast amounts of structured and unstructured data seamlessly. Advanced indexing and metadata management will improve accessibility and usability.
5. AI-Powered Automation Tools
Automation tools enhanced by AI will further streamline repetitive tasks, such as compliance monitoring, anomaly detection, and resource allocation. This will free up human resources for more strategic initiatives.
6. Sustainable AI Practices
As businesses aim for greener operations, sustainable AI will focus on reducing energy consumption in AI model training and deployment. Cloud providers will prioritize eco-friendly data centers and energy-efficient algorithms.
By staying ahead of these trends, businesses can ensure their cloud data management strategies remain robust and innovative. The future holds immense potential for AI and ML to redefine how data is managed, analyzed, and leveraged for growth.
Conclusion
AI and ML are no longer just tools of the future—they are reshaping the present and setting the stage for unprecedented innovation in cloud data management. By leveraging these technologies, businesses can automate complex tasks, enhance security, and derive actionable insights, all while optimizing costs and improving scalability.
However, success in this realm demands a strategic approach. It requires organizations to address challenges such as data quality, skills gaps, and compliance concerns while embracing best practices for integration. Additionally, staying informed about emerging trends like explainable AI, federated learning, and edge computing will ensure businesses are not just reactive but proactive in their data management strategies.
Why Businesses Trust SecureMyOrg for Comprehensive Network Security
At SecureMyOrg, we uncover and fix all possible security vulnerabilities of mobile and web, while providing solutions to mitigate risks. We are trusted by renowned companies like Yahoo, Gojek and Rippling, and with 100% client satisfaction, you’re in safe hands!
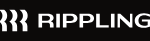

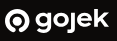
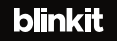
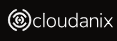
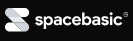
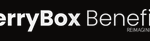
Some of the things people reach out to us for –
- Building their cybersecurity program from scratch – setting up cloud security using cost-effective tools, SIEM for alert monitoring, building policies for the company
- Vulnerability Assessment and Penetration Testing ( VAPT ) – We have certified professionals, with certifications like OSCP, CREST – CPSA & CRT, CKA and CKS
- DevSecOps consulting
- Red Teaming activity
- Regular security audits, before product release
- Full time security engineers.
Relevant Posts
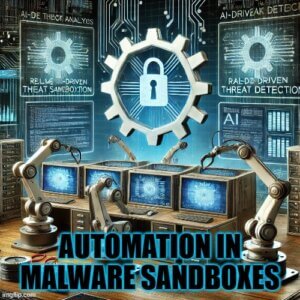
Automating Threat Intelligence with Malware Sandbox Solutions
As cyber threats become more sophisticated, manual threat analysis is no longer sufficient. Automated malware sandbox solutions offer real-time detection, seamless integration with threat intelligence platforms, and enhanced incident response. By leveraging AI and behavioral analysis, these solutions help organizations stay ahead of evolving cyber threats.
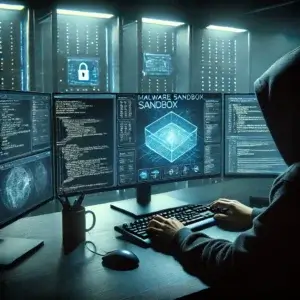
How to Set Up a Malware Sandbox for Effective Threat Analysis
Setting up a malware sandbox is essential for analyzing and mitigating cyber threats in a secure environment. This guide walks you through the step-by-step process of creating an effective sandbox, from choosing the right virtualization platform to configuring security tools and evasion resistance techniques.
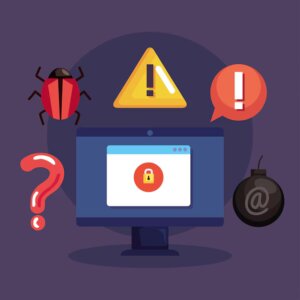
Best Malware Sandboxes in 2025: Top Tools for Security Analysts
Malware sandboxes play a crucial role in cybersecurity by providing a safe environment for analyzing malicious software. In 2025, several top-tier malware sandboxes, including Cisco Threat Grid, FireEye AX, VMRay Analyzer, and Cuckoo Sandbox, offer powerful detection, evasion resistance, and automation capabilities. This blog explores the best malware sandboxes of 2025, highlighting their key features and helping security analysts choose the right tool for effective threat analysis.
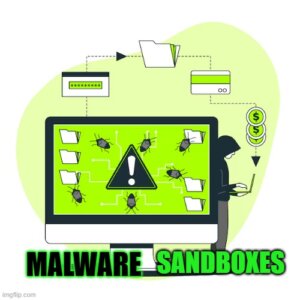
Understanding Malware Sandboxes: How They Work and Why They Matter
Malware sandboxes are a crucial tool in modern cybersecurity, allowing security professionals to analyze, detect, and neutralize malicious software in a controlled environment. By executing suspicious files in an isolated setting, sandboxes help uncover hidden threats, detect advanced malware, and enhance threat intelligence. In this blog, we explore how malware sandboxes work, their types, and why they are essential for safeguarding digital assets against evolving cyber threats.
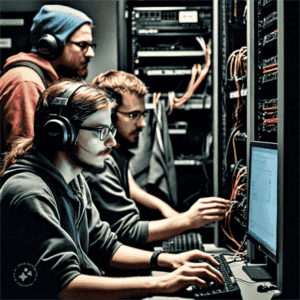
Ethical Hacking vs. Penetration Testing: What’s the Difference?
Discover the key differences between ethical hacking and penetration testing. Learn how these cybersecurity practices complement each other to safeguard your organization against evolving threats.
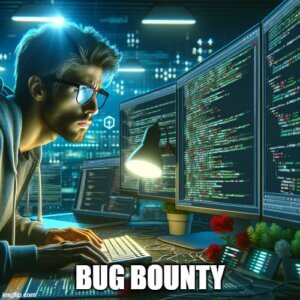
Bug Bounty Programs: A Lucrative Opportunity for Ethical Hackers
Bug bounty programs offer ethical hackers a unique opportunity to earn rewards by identifying and reporting security vulnerabilities. With major tech companies and organizations investing in cybersecurity, these programs have become a lucrative career path for skilled hackers. In this blog, we explore how bug bounty programs work, the skills required, and tips to maximize earnings in this competitive field.